- Point of view
How to harness the transformative growth of generative AI in F&A – Part 1
Key considerations and risks to overcome for CFOs
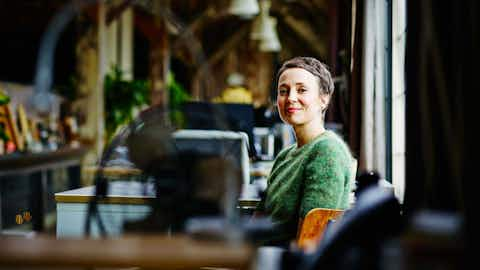
The rise of generative AI has been intense and continues to fill headlines and discussions across industries and functions. From helping pharmaceutical companies discover tomorrow's blockbuster drugs to being a record label's next big signing, gen AI is being discussed in both board rooms and at dinner tables. And finance leaders are not immune.
This rapidly evolving technology has redefined the potential for intelligent automation technologies and galvanized businesses to make a groundbreaking shift in their operations and strategies. CFOs are also wading into the generative AI waters, given its pivotal role in an organization's digital transformation and decision-making.
In the first of this two-part series, we explore how CFOs can realize the benefits and factors they need to succeed and deliver value.
What is generative AI?
Generative AI is the umbrella term for the subset of artificial intelligence that uses large language models (LLMs) – a type of AI algorithm that can process vast volumes of text – to identify patterns in existing data to create new, seemingly original content. It can produce text, images, audio, synthetic data, and more. For finance and accounting (F&A), that means rethinking how teams work to accelerate cycle times, better serve internal and external customers and suppliers, and gain faster access to insights for sharper decision-making.
We see a future in which finance executives will be able to send text- or voice-based commands to LLMs to trigger approvals, send alerts, generate custom key performance indicators, close disputes, and more. An LLM will become an invaluable personal assistant to finance teams.
Injecting greater insight into intelligent automation
For many finance leaders, end-to-end process automation has been a long-awaited goal, connecting functions across their businesses. Now, with generative AI complementing their existing digital landscape, finance teams will push the boundaries of process automation, find new ways of working, and enable greater collaboration and efficiency.
There is immense enthusiasm among finance professionals to quickly adopt this technology. Generative AI is now top of mind for many corporates. We'd say that 90% of tech service providers highlighted how they're creating generative AI models in their Q1 2023 earnings calls.
F&A tech integrators see the value of generative AI in finance from a software-as-a-service (SaaS) standpoint, and many have announced new offerings and use cases to advance its adoption. For example, SAP has partnered with Microsoft to offer recruitment solutions, Salesforce unveiled Einstein GPT, ServiceNow launched StarCoder, and Oracle has healthcare-specific use cases. This will only intensify, so companies must be ready to filter out hype from reality.
Take a copy for yourself
Generative AI-powered F&A
As we look across digital operations in finance, we see generative AI use cases fall into one of three categories:
- Processing and summarizing large volumes of content in a flash
- Generating text-based content and graphics
- Interacting with other systems or applications to execute workflows faster than ever
We believe that generative AI/LLMs will have the greatest impact in the following areas:
- Accounts payable (AP) helpdesks and customer service: this is where generative AI will make its biggest mark – by generating responses for queries and resolving general as well as complex issues for vendors and suppliers
- Invoice to cash: integrating chatbots with SaaS will improve experiences and generate new insights, creating outcomes that go beyond productivity
- Financial planning and budgeting: generative AI is dramatically changing how teams write commentary, search for insights, and collaborate. Previous low levels of accuracy using existing natural language processing (NLP) solutions will rise significantly
- Enterprise risk management: the technology will improve risk assessments by seamlessly incorporating additional factors and attributes like geopolitical and supply chain concentration risks at speed
We explore these use cases in more detail in part two of this short series. But before CFOs can realize the benefits of generative AI in finance, they must first understand what they need to do to succeed with the technology.
Enhancing finance processes
Not every business process will fall into one of the use-case categories. So, finance teams need a robust framework to evaluate the extent to which LLMs and generative AI can automate a business process.
An LLM learns patterns and structures from large amounts of data upon which generative AI is based. To work with them most effectively, companies need to consider whether they have the right tech capabilities, strong data foundations, and a robust digital ecosystem to create a successful LLM that offers contextually relevant results.
These four factors will determine whether an LLM can enhance a business process:
- Tech capabilities: deciding whether an LLM model can fully or partially take up a task will depend on the following generative AI capabilities:
- Text conversation: human-like conversational capabilities like a chatbot service. For example, a vendor helpdesk chatbot or a digital collection agent
- Text classification: text extraction and classification of information within a specific context. For example, extracting key data from legal contracts like lease, revenue, royalty, or commission data
- Information search: document-based search and insight generation. For example, for query generation and code searches, replacing self-service reporting with human-like query-based insight searches
- Content generation: summarizing, translating, and generating text. For example, when writing emails, letters, or code, generative AI can write commentary for variance analysis, board reports, financial reporting, earnings calls, and other types of reporting
- Data and training effort: The data a generative AI model is trained on will determine its success or failure, so finance leaders must understand the effort required to build and maintain the data and tech for an LLM-led solution. These models depend on the queries and responses they are trained on and whether they are diverse and comprehensive. They must also be trained on how to handle and avoid inaccurate responses.
With inadequate training, LLMs can generate hallucinations: responses that the model appears confident are accurate but may be factually incorrect. So, the datasets and feedback used to train generative AI models need to be carefully curated to create accurate content with the right context. - Digital ecosystem: The generative AI buzz is not set to die down anytime soon, and many business leaders are pursuing different applications. But the reality is that generative AI is just another digital tool and another part of a company's digital strategy.
Enterprises need to carefully evaluate whether the business problem at hand is better solved by an existing digital solution or whether generative AI can work in tandem with other technologies to increase the degree and pace of automation. We're already seeing a variety of tech partnerships, such as AWS, Google, and Microsoft, that are integrating LLMs in companies' digital models. - Regulatory concerns: Today's generative AI models have been trained on massive amounts of data. For enterprises, it's critical that they can trace and track the output generated to either proprietary data owned by the enterprise or the rightful IP owner. This is especially important considering countries are also evolving their regulations on IP rights, data privacy, and security.
Overcoming the risks of adoption
Generative AI holds great potential, and many finance leaders are building solutions that can deliver trusted outcomes. But there are risks. In addition to producing hallucinations, bias can creep in if models are trained on data that reflects prejudice. And if it holds personal or sensitive information, it could lead to unethical decisions and the misuse of personal information. This is why maintaining a human in the loop is essential. People can spot bias and bring the necessary context and experience that enable confident decision-making with generative AI.
As more countries introduce regulations to protect intellectual property and data privacy and security, enterprises must build responsible generative AI practices and governance that lead to responsible decision-making. Finance teams can build iterative prompt frameworks by exposing the model to many sample scenarios, testing the results thoroughly, and building an internal governance mechanism to overcome potential risks.
At Genpact, we've built guiding principles around our AI initiatives that govern our actions and enable us and our clients to use generative AI responsibly. For example, we use a privacy-by-default framework that allows organizations to run due diligence and enhance transparency. Our approach protects AI systems, applications, and users while also expediting the pace and scale of software development.
But, adopting a responsible AI framework is not enough. Leading CFOs are also creating awareness and building cultures of responsible AI as a core value across functions.
What's next?
By embracing the benefits gen AI holds and understanding the effort and risks involved, organizations can navigate the technology's complexities and ambiguities, forging a clear roadmap for adoption. This is how enterprises can drive scale and deliver outsized impact.
In part two, we explore the prominent gen AI use cases for finance in more detail – looking beyond productivity and cost savings. Gen AI is empowering helpdesk capabilities, building customer loyalty, accelerating decision-making, and paving the way for digital-led finance transformation.