- Point of view
Responsible AI: Developing a framework for sustainable innovation
Building ethical products and services for everyone
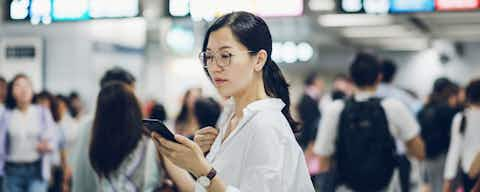
Artificial intelligence (AI) is drastically changing how we live and work. From prioritizing patients for emergency medical care to checking employment and housing eligibility or even selecting the information we view on social media, the use of AI is everywhere. Yet, rooting out potential biases like gender and race discrimination remains a significant challenge.
At its core, AI aims to replicate human intelligence in a computer or machine with greater accuracy and speed. And over the next decade, AI's influence could exponentially grow as enterprises deploy the technology in banking, transportation, healthcare, education, farming, retail, and many other industries. Research firm Fortune Business Insights predicts the global AI market could reach $2.74 trillion by 2032 from $621 billion in 2024, an increase of 341%.
But as AI becomes a central part of society, concerns around its ethical use have taken center stage. For the enterprises that fail to act, legal and reputational consequences could follow. Here, we'll review the responsible AI challenges and how to overcome them.
Concerns behind the growing role of AI
The lack of clear ethical guidelines in AI and machine learning (ML) systems may have several unintended consequences for individuals and organizations (figure 1).
Potential risks of AI
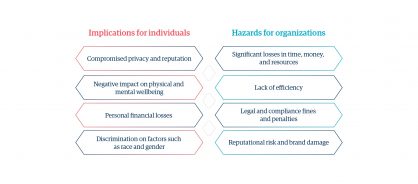
Enterprise leaders cannot ignore these risks because the potential damage to people and organizations can prove significant, as these two examples show:
- In 2019, Apple's credit card processing practices came under fire for gender discrimination. Danish entrepreneur David Heinemeier Hansson received 20 times more credit than his wife, even though they filed joint taxes and she had a better credit history. [1]
- The same year, the US National Institute of Standards and Technology (NIST) found that facial recognition software used in areas like airport security had trouble identifying certain groups. For example, some algorithms delivered significantly higher rates of false positives for Asian and African American faces relative to images of Caucasians. [2]
These are just a couple of examples, among many others, that contributed to a global debate around the use of AI, prompting lawmakers to propose strict regulations. A lack of trust has forced AI practitioners to respond with principle-based frameworks and guidelines for the responsible and ethical use of AI.
Unfortunately, these recommendations carry many challenges (figure 2). For example, some enterprises have developed insufficient or overly stringent guidelines, making it difficult for AI practitioners to manage ethics across the board. Or even worse, other organizations have inadequate risk controls because they don't have the expertise to create responsible AI principles.
At the same time, algorithms are constantly changing while ethics vary across regions, industries, and cultures. These characteristics make rooting out AI biases even more complex.
Challenges in responsible AI/ML development
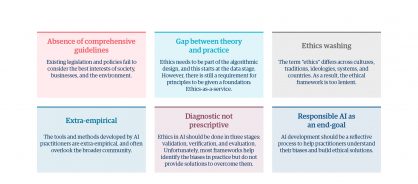
A new approach: The responsible AI center of excellence
To develop AI solutions that are fair, trustworthy, and accountable, enterprises are building AI centers of excellence that act as ethics boards. By connecting diverse groups of AI custodians – who can oversee the development of artificial intelligence from start to finish – enterprises can proactively prevent issues down the road. Put simply, teams of people with different experiences, perspectives, and skill sets can catch biases upfront compared to homogenous groups.
Thankfully, with a practical framework in place (figure 3), AI practitioners can establish ethics-as-a-service to assist and guide organizations through AI implementation and development.
Responsible AI in practice
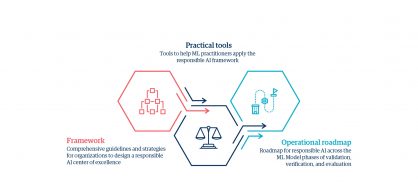
The four pillars of a responsible AI framework
Developing a comprehensive framework is the first and most crucial stage of a responsible AI journey, and it usually consists of four pillars:
1. Governance body
Enterprise leaders must establish an AI/ML governance body (figure 4) of internal and external decision makers to oversee the responsible use of AI continuously. In addition, C-suite executives should prioritize AI governance – other business leaders can then be held accountable for developing governance policies alongside regular audits.
Responsible AI governance body
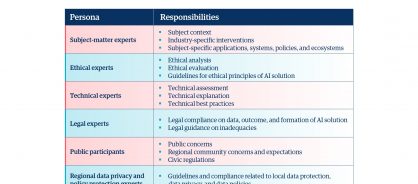
2. Guiding principles
Transparency and trust should form the core principles of AI. We've identified seven characteristics to help enterprise leaders form a strong foundation for safe, reliable, and non-discriminatory AI/ML solutions:
1. Domain-specific business metrics evaluation
2. Fairness and legal compliance
3. Interpretability and explainability
4. Mitigation of changes in data patterns
5. Reliability and safety
6. Privacy and security
7. Autonomy and accountability
8. Traceability
Similarly, a governance body can help enforce these principles throughout the development of AI technology (Figure 5).
Matrix of AI governance body
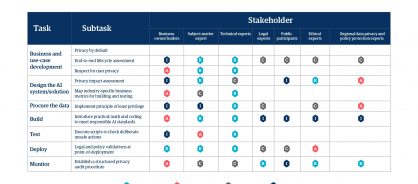
3. Realization methodology
AI solutions will inevitably touch multiple areas of the organization. As a result, enterprise leaders must account for and involve all stakeholders, from data scientists to customers. This process also requires having clear risk controls and a framework for responsible AI (figure 6).
Realization methodology for Genpact's responsible AI framework
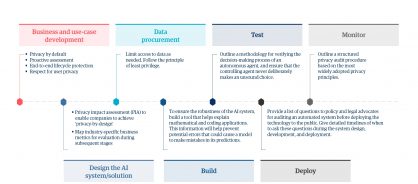
4. Implementation overview
The inherent complexities in developing AI algorithms make interpreting models challenging. However, enterprise leaders can embed responsible AI considerations throughout the process to help mitigate potential biases and follow best practices (figure 7).
Genpact's responsible AI framework implementation
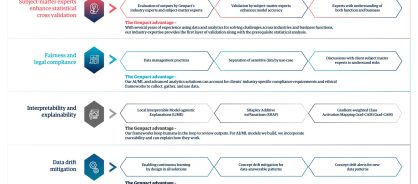
Case Study
A global bank puts responsible AI theory into practice
A bank wanted to streamline loan approvals while removing potential biases from its loan review process. As a first step, Genpact improved the bank's data management and reporting systems. Then, with a robust data taxonomy in place, we applied our responsible AI framework. For example, removing variables like gender and education increased the probability of reaching a fair decision. We also improved the quality of the reports, helping employees enhance transparency by showing the data behind their decisions. Finally, we developed a monitoring system that could alert the AI ethics board of any potential issues. The success of this project has led the bank to deploy similar AI ethics models across the organization.
Embracing the responsible AI opportunity
Customers, employees, partners, and investors increasingly expect organizations to prioritize AI ethics to build safe and reliable products. With a responsible AI framework in place, organizations can continue innovating, building trust, and increasing compliance. These benefits will help organizations sustain long-term growth, improve competitive advantage, and create value for all.
AI helps enterprises make informed and responsible decisions. Discover how data-driven sustainability influences financial, social, and environmental change.