- Blog
Three ways AI is transforming data management
Explore how tailored AI solutions address data issues and enable AI at scale
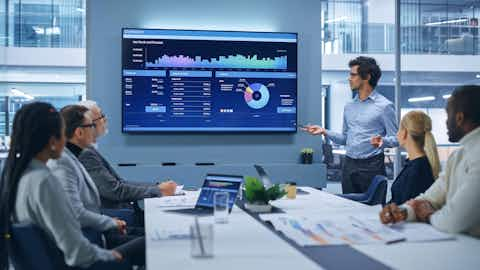
Remember the tale of "The Three Little Pigs" and the Big Bad Wolf? The wolf huffs and puffs, easily blowing down the houses made of straw and sticks. But the brick house stands strong, saving the pigs from danger.
Not surprisingly, enterprise leaders are finding their data foundations to be as flimsy as straws or sticks. Such frail structures have very real adverse repercussions on companies' ability to harness the benefits of artificial intelligence (AI) at scale.
Case in point: While executives acknowledge that robust data strategies are essential for unlocking AI's full potential, only 16% of enterprise leaders surveyed in HFS and Genpact's generative AI (gen AI) report are using proprietary data for gen AI initiatives.
These anemic numbers are symptoms of underlying challenges organizations face, including:
- Disconnected applications from outdated systems, resulting in scattered data sources
- Lack of a single source of truth makes it hard for teams across departments to find trusted data
- Poor data quality hampers decision-making and leads to unreliable insights
- Data silos create friction and inefficiencies among teams and functions
The good news is we can change this narrative. By starting small and using targeted AI solutions that show tangible value, organizations can build confidence and momentum toward broader AI implementation at scale. Here are three steps to help you get started:
How AI can enhance data quality
In our gen AI study, 42% of IT leaders identified a lack of data quality or strategy as their primary impediment to effectively employing gen AI.
Good data builds a strong AI foundation. It should be complete, accurate, relevant, consistent, and readily available. You cannot afford missing metadata, duplicates, or other issues that undermine quality.
Here are a few ways AI can help boost data quality:
- Enriching data catalogs: AI can analyze unstructured data, like images and artwork, to improve product details and enhance catalog completeness and searchability
- Cleansing datasets: AI and machine learning can standardize attributes and predict missing fields, enriching datasets by filling in gaps in information
- Anomaly detection: AI can identify and remove duplicates, detect outliers, and analyze trends within datasets, improving AI data quality and integrity
How AI can improve data lineage
Effective AI data governance starts with understanding where data comes from. This is why data lineage is critically important.
Key to that goal: a technical catalog and a well-structured data dictionary, which clarifies what the data represents and the value it can deliver.
AI can help data lineage by:
- Conducting root cause analysis: AI can quickly pinpoint the exact source of data errors – such as broken integration processes or incorrect data entry – making it easier to trace issues when data goes awry
- Enhancing discoverability: As data moves within and outside of tech stacks, AI can track its movement in real time, helping organizations understand the data life cycle
- Bolstering compliance: AI data lineage tools can generate necessary documentation to meet compliance requirements related to data sovereignty and other laws, saving valuable human resources
How AI can optimize data architecture
Data architecture determines how teams manage and store information.
For some time, organizations have struggled to create a centralized platform for all data to flow into while trying to integrate federated or distributed data sources. The alternative has been to rely on point-to-point solutions, which lead to bloat and the inefficiencies associated with siloed systems.
AI can help data teams bypass these challenges by creating a knowledge graph that integrates data sources and optimizes AI data processing. As a key component of an intelligent data platform, the knowledge graph allows users to access use-case recommendations and gain valuable insights by querying the AI in plain language.
AI can optimize data architecture by:
- Creating knowledge graphs: AI can weave together disparate data sources, enabling seamless integration and access
- Delivering use-case recommendations: The intelligent data platform can provide insights into how to best use data for various business needs
- Facilitating plain-language queries: Users can easily access valuable insights without needing deep technical expertise
One example of this is Genpact's PowerMe, which enables organizations to discover, trace, and trust their data by improving data quality, tracking lineage, and delivering an intelligent data catalog.
Futureproofing your data
Looking ahead, as generative AI use cases multiply – enhancing data quality and democratizing access to insights – organizations can learn from the tale of "The Three Little Pigs." By adopting tailored solutions to address specific data challenges, they can build a solid, brick-like foundation for AI data management. This strong base not only withstands the huffing and puffing of evolving data demands but also paves the way for enterprises to scale AI effectively and unlock its full transformative potential.