- Blog
Three AI predictions for 2025
Here's what to expect as AI takes firmer root in enterprises
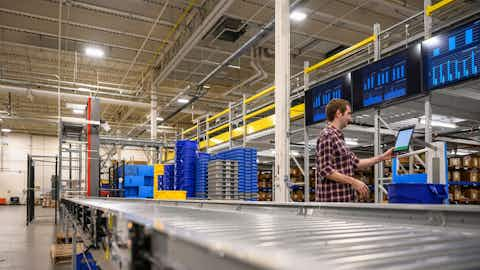
If 2023 marked AI's breakout moment, 2024 became the year it took root, finding applications across nearly every industry. Yet a significant gap persists between AI's immense potential and its execution. According to the HFS and Genpact generative AI report, 95% of companies have yet to achieve generative AI maturity. With mounting pressure to keep pace with innovation, enterprises are rapidly accelerating their AI adoption and scaling initiatives.
As enterprise leaders refine their strategies, here's where I see AI heading in 2025.
Agentic AI has the potential to flourish
Unlike generative AI, agentic AI doesn't just analyze data or make recommendations – it takes action to carry out decisions. As a result, agentic AI systems provide end-to-end automation, going beyond handling isolated tasks to create continuous, integrated workflows that operate seamlessly across different processes.
Unleash agentic AI on efficient workflows, and it becomes a solution that operates 24/7. Take day trading, for example, where transactions execute autonomously when market conditions align. Unlike traditional algorithmic trading, which follows predefined rules, agentic AI adapts in real time, continuously refining strategies as market dynamics change.
Despite the limitations of agentic AI, organizations are already deploying AI agents. The biggest challenge? AI agent development suffers from a lack of dedicated infrastructure. However, as use cases solidify, both developers and users will seek platforms that can enhance the performance, reliability, and scalability of agentic AI.
Expect agentic AI to gain significant traction in 2025, particularly in AI-mature enterprises.
Multimodal AI gains momentum
Data scientists trained early generative AI models primarily on text. With multimodality, they are expanding their focus to include images, video, and sound.
Take insurance claims, for example. A multimodal AI system can process PDFs, scanned images, and emails. It can train large language models to classify the documents, extract key fields such as claim numbers and customer information, and summarize the context of the claim. If the claim involves audio, the system can also transcribe and analyze the data, including performing sentiment analysis to assess the customer's emotional tone and urgency.
The multimodal system also handles the complex task of sifting through long email chains, extracting key updates, actions, or decisions, and determining the status of each claim. With multi-agent capabilities, the system can then generate a conversation timeline, flag missing documents, and suggest or execute next steps, such as automatically requesting additional information or escalating the case for human review.
Organizations already have tons of video and image data, and with multimodal AI, they can finally make the most of it.
I'm particularly excited about the potential impact on businesses. With multimodal AI, we could see stronger supply chains where warehouse employees scan and catalog products to create more comprehensive repositories. Meanwhile, fraud detection and customer service teams can uncover significant opportunities by integrating voice, image, and text data into existing workflows.
Responsible AI continues to take center stage
AI regulations remain fragmented worldwide, creating a complex and unpredictable environment for developers. In response, many companies focused on responsible AI are developing proprietary governance modules tailored to different regions. This approach helps organizations stay ahead of evolving regulations, ensuring their systems remain ethical and compliant.
I've spoken about responsible AI before, but it bears repeating because the stakes keep rising. After all, we are entering an era of agentic, multimodal AI. Next year will mark a turning point, with the establishment of robust frameworks for AI governance setting the stage for safer and more accountable systems as AI expands into more use cases.
With regulatory demands like the EU AI Act requiring explainability, responsible AI has become crucial for ensuring compliance and mitigating operational risks. It also plays a vital role in driving continuous improvement and boosting stakeholder confidence.
To achieve this, organizations must build strong governance frameworks, define clear goals aligned with business objectives, adopt tailored tools that optimize performance and scalability, and integrate explainability into the AI life cycle. This integration is essential for long-term success.
By 2025, I predict that industries and governments will collaborate to create unified AI governance frameworks that balance ethical standards with innovation. This collaboration is essential to avoid regulatory fragmentation that could stifle progress and undo years of development.
Scaling AI in 2025 and beyond
As organizations face challenges scaling AI, 2025 may see increased efforts to build curated, high-quality datasets that enhance model performance and address these challenges. These initiatives will rely on human expertise to ensure the delivery of reliable and accurate model output.
AI has already delivered impressive results in 2024, streamlining processes, eliminating bottlenecks, and boosting efficiency.
In 2025, enterprise leaders could move beyond isolated wins to scale their AI initiatives for broader impact. With agentic AI driving seamless automation, responsible AI ensuring ethical practices, and multimodal AI unlocking new ways to harness data, we are entering the next phase of the tech revolution.